Related posts
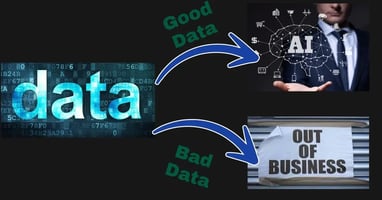
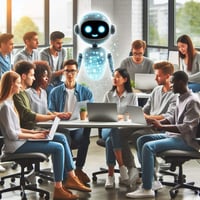
Let’s dive in and explore how AI can help developers or engineers be more productive, more...
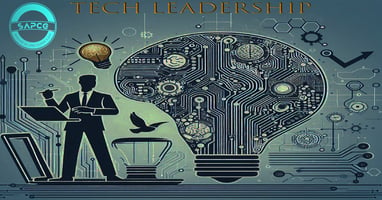
“But this is how we’ve always done it.” Have you heard that before? In many technology...
Let’s dive in and explore how AI can help developers or engineers be more productive, more...
“But this is how we’ve always done it.” Have you heard that before? In many technology...
Sometimes, the return on investment (ROI) of AI requires some patience but trust me the ROI is there with AI. While there are plenty of quick wins with AI, which we will cover in the next post, this one focuses on the long game. Specifically, we’ll explore what it takes to see ROI on larger projects and how they align with strategic planning for your organization.
A Common Use Case
Let’s examine a common use case where a company, PayLnk, wanted to leverage their extensive product documentation to answer clients’ questions on how to use their software. PayLnk had previously attempted to implement this using traditional search, but they found the results too limited by needing to use exact phrases, often leading to incorrect results. This was further proven through customer satisfaction surveys that highlighted one of the biggest pain points: finding the right documentation quickly.
The Importance of a Data Strategy
As discussed in the previous post, “What’s Wrong with My AI? The Importance of a Data Strategy”, we first need to examine the data strategy to ensure we have the data, and it’s properly controlled. Fortunately for PayLnk, they have very detailed documentation and controlled processes in place to keep it up to date and accurate. So, can AI improve their client experience for finding the right documentation quickly? The answer is yes. Let’s explore why that is and how to solve the problem, at least from a 10,000 ft level.
The Bedrock of the Solution
The foundation of this solution will be:
Vector Search
The engine needs the ability to infer likeness, or what we call in the AI space vector-based searches, where it can take search terms and apply conceptual likeness. Conceptual likeness allows the user to enter multiple terms but get the same results. For instance, if you have a company standard of using “Feline” in all your documentation, you want users to be able to enter terms such as “cat”, “feline”, “kitty” or “moggy” and all return results for “Feline”. AI engines such as OpenAI and Azure OpenAI can natively handle this, eliminating the need to create a library of synonyms for traditional search.
Vector Indexing
Vector indexing is the process of taking your data and metadata and applying numerical arrays to the data for fast retrieval. This topic is extremely complex, and it’s important to pick the right index strategy for your data and use case or the right software vendor that already knows this world and can set you up for success.
Application Integration
This is the final step in delivering your solution to your users. Make sure the solution you choose has an easy-to-implement API or SDK. However, be aware of solutions that are proprietary or have a heavy uplift on cost that is activity or per search based that can get expensive quickly. Make sure to fully understand the pricing model as this will impact your ROI.
Evaluating ROI
Achieving a highly positive ROI will be a test of patience and dedication to AI being part of your long-term solution. In PayLnk’s case, they learned that while their documentation was solid, it wasn’t necessarily built for AI. They had to make several changes to their metadata and documentation formatting to make it more readable and accurate for vector searches. It took about 3 months and about 20 updates to their documentation standards to achieve a 97% first search match rate and turn the capability over to their customers directly.
The total cost of implementation for this was $75,000 annually for the third-party software that provides the indexing and search capabilities, and $280,000 of people costs to test, implement, and make documentation changes. The changes have led to a drop of approximately 330 support tickets a month at an average employee cost of $40 per ticket or $13,200 a month in people savings. Not taking into consideration the number of new customers or customer retention, the timeframe to get net positive on the investment is about 3 years and 3 months. This is where the patience comes in and also data tracking becomes important as if you track in the sales and retention process the reason for closing deals or retaining a client and they give the improved documentation and search the ROI can be realized much earlier.
If all this has you spinning your wheels, then reach out to SAPCG and we will be happy to help you navigate this landscape.
Contact us at info@sapcg.co